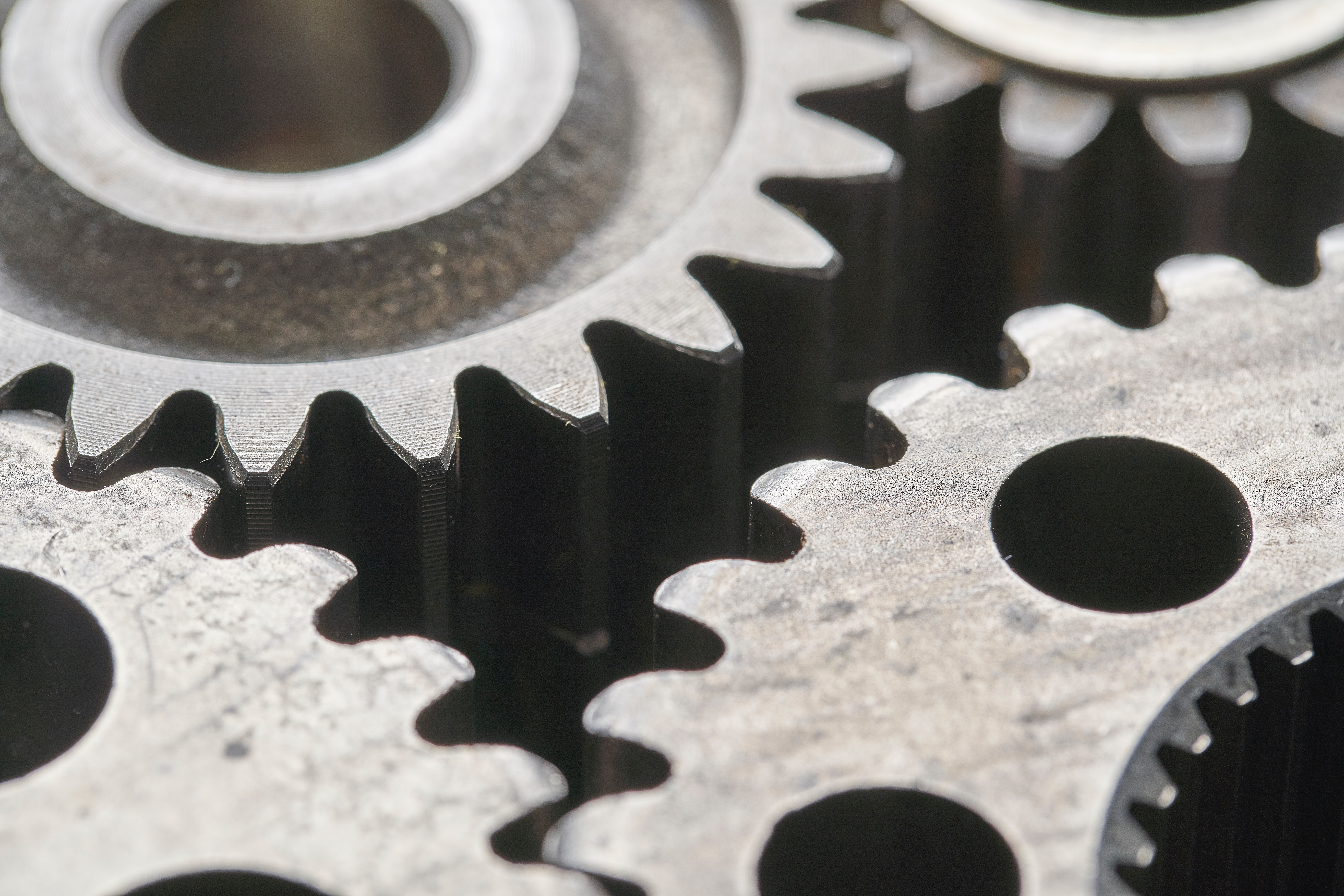
While many companies have embarked on data analytics initiatives, only a few have been successful
While many companies have embarked on data analytics initiatives, only a few have been successful. Studies have shown that over 70% of data analytics programs fail to realize their full potential and over 80% of the digital transformation initiatives fail. While there are many reasons that affect successful deployment of data analytics, one fundamental reason is lack of good quality data. However, many business enterprises realize this and invest considerable time and effort in data cleansing and remediation; technically known as data engineering. It is estimated that about 60 to 70% of the effort in data analytics is on data engineering. Given that data quality is an essential requirement for analytics, there are 5 key reasons on why data analytics is heavy on data engineering.
1.Different systems and technology mechanisms to integrate data.Business systems are designed and implemented for a purpose; mainly for recording business transactions. The mechanisms for data capture in Business systems such as ERP is batch/discrete data while in the SCADA/IoT Field Systems it is for continuous/time-series data. This means that these business systems store diverse data types caused by the velocity, volume, and variety dimensions in the data. Hence the technology (including the database itself) to capture data is varied and complex. And when you are trying to integrate data from these diverse systems from different vendors, the metadata model varies resulting in data integration challenges.
2. Different time frames of data captureThe timeframes for data ingestion during data capture varies. For example, in ERP/transactional systems the data ingestion is typically batch/discrete/manual, while in SCADA/IoT/Field Systems, the data ingestion is usually automatic and real-time. For example, when the product delivery to the customer is done, the shipment details are normally captured in real-time by the hand-held devices. But the invoicing cannot be immediately processed as invoices are issued from the ERP systems to the customer. This creates a delay in Delivery-Invoicing compliance reporting.
3. Different user value-propositionsIn business, the same data is created and consumed by different stakeholders (inside the company) in different ways as their value-propositions vary. For example, vendor payment terms for Finance is a cost object, while for Procurement the same data element is a risk element (as longer payment terms generally result in longer deliveries).
4. Different business processesThe same data element can be different due to differences in business processes based on geographies, laws, regulations, market conditions, etc. For example, the Date-of-Birth data element in Canada is subject to data privacy regulations, while Date-of-Birth data element in most developing countries is generally not part of the data privacy regulations. So, getting customer buying habit report based on age for a developing market is much "easier" than getting the same report in Canada.
5. Different aggregations driven by organizational structuresOne data element can be viewed differently based on differences in granularities or aggregations driven by organizational structures. For example, the VP of procurement might need a spend report based on item categories (an aggregation of items procured), while the procurement manager needs the spend report based on individual items procured. That aggregation might vary based on the item type, supplier type, delivery location, etc.
Good analytics relies on good quality data. So, if you are embarking on the analytics journey by looking at technologies, tools, and hiring data scientists, pause for a minute. Challenge your assumption and ask one basic question – is diversity of my business operations affecting a good quality data for analytics? If the answer is yes, get ready for a long and a complex data engineering effort.